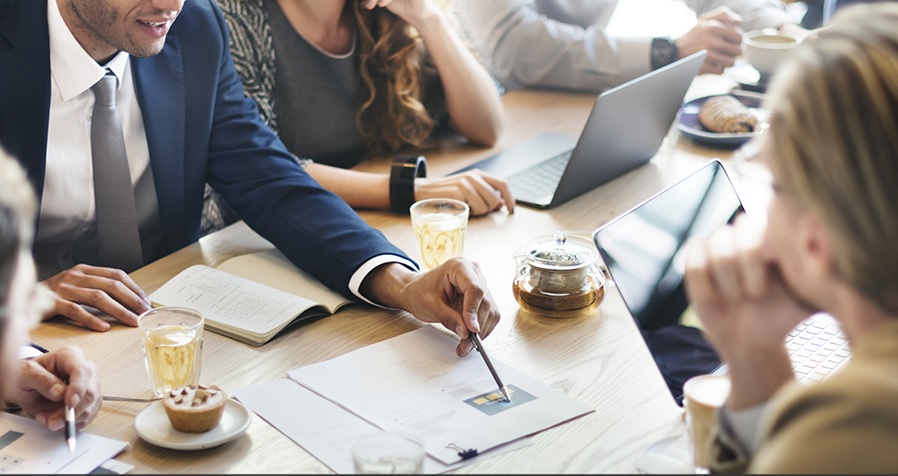
Organizations are beginning to realize that profits and opportunities are lost when they have neglected their data and have corrupt, incomplete, non-standardized or replicated data. Inaccurate data wastes exponentially more time to fix after the fact than if it had been fixed when it was first collected. According to Forbes, poor data quality also harms your ability to perform analytics that can be trusted in order to make short- and long-term business decisions. Poor data can result in lost sales and frustrated customers.
What is Data Quality? –
Simply put, Profisee explains that you have high quality data when you can glean from the data that which you desire, for example for marketing analytics. Also, high quality data shows a picture of what is occurring in your interactions with your customers accurately.
Some Important Definitions –
As in any aspect of business, there is some jargon that you will need under your belt in order to understand data quality management:
GDPR: If you are not from the EU, this acronym might not be familiar. It is a 2012 set of guidelines that businesses in the EU must follow in order to provide data protection. The acronym stands for “General Data Protection Regulation.” One huge issue in the quality of data is meeting regulatory compliance rules in order to avoid huge fines. Also, as we are all well aware, data breeches can be expensive for businesses – both in monetary terms and in terms of loss of reputation and customer churn.
Churn: This is a word that we weren’t so much using in this context even a few years ago. Customer churn has come to mean when a customer no longer wishes to use your company for their product or service needs. Churn ratio means the number of customers that leave your company in a given time period over those that have stayed loyal. High quality data helps companies understand the “whys” behind customer churn, so you can hopefully decrease this ratio and possibly get back those customers that have already broken ranks.
Data Quality KPIs: According to Profisee, good Key Performance Indicators (KPIs) for the quality of your data include the percentage of replicated customer files. Other KPIs for data assess if the data is complete, accurate, standardized, precise and up-to-date.
Data Profiling: Data profiling is the technique used to determine the DQ KPIs – looking for robust records that are complete, error-free, standardized, necessary and singular.
Data Matching: Data matching helps to find the same person or business across one or more databases with the goal of merging duplicate records.
Data Governance: Data governance manages all aspects of data and its use in an organization, including a data management team and leader, an agreed upon set of rules for the data and plans for the governance, cleansing and repair of data.
Tips on Improving the Quality of Your Data –
Information Week suggests some initial tips on moving towards higher quality data:
- Hire a DQ Manager and create a DQ team comprised employees from the different departments in your organization
- Have this group decide which data is needed and which is extraneous
- Create standardization rules and constraints on how data is input, so it will be accurate from the start
- Use data cleansing software regularly
- Make it a point to update customer data often
Businesses that make the collection and maintenance of high-quality data a priority will then be able to move to the next step of using business analytics to determine how to keep their present customers happy and find new converts. Also, they will avoid costly mistakes, such as regulatory non-compliance issues and failing to address small problems that can fester and grow.